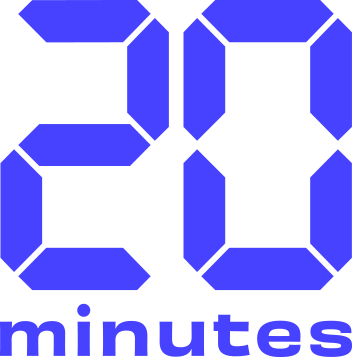
20 Minutes: Turning cloud savings into news innovations
20 Minutes reassigns two full-time engineers to new projects with the help of DoiT
Automatically manage cloud compute for optimized costs and agility
Make sense of spend and chargeback to align with your business
Optimize BigQuery costs with actionable recommendations and usage insights
Maximize AWS Spot savings and minimize disruptions for optimized scaling
Autonomously identify cost spikes early, with zero configuration
Ingest third-party data to see combined analytics in a single pane of glass.
Organize your billing data for better business decisions
Connect your favorite tools to DoiT’s products
Learn how we’re redefining support with our customer reliability engineering
View our live support and customer satisfaction statistics in real-time
Proven solutions to cloud complexity
A global team of committed cloud experts with decades of experience in cloud architecture, Kubernetes, machine learning and much more
Learn how DoiT enables critical FinOps capabilities
DoiT Accelerators give you the dedicated cloud expertise and resources to quickly adopt new cloud services and build production-ready workloads with them.
Implement end-to-end Gen AI solutions into your product with Amazon Bedrock and more
Accelerate new EKS deployments
Develop a production-ready, modern data platform on AWS
Implement end-to-end Gen AI solutions into your product with Vertex AI and more
Accelerate new GKE deployments
Develop a production-ready, modern data platform with BigQuery and beyond
Proud to be an award‒winning multicloud partner to top‒tier cloud providers
Enabling cloud growth and unlocking revenue through expert partnership
Accelerate new customer growth and Marketplace integration on AWS and GCP
Read the latest insights, tips and perspectives from our team of cloud experts
See how we’ve helped thousands of public cloud customers achieve their goals
Listen to our experts and customers share tangible tips for navigating the cloud.
Tech talks and interactive expert sessions delivered both virtually and in person
Discover foundational expertise and future-ready recommendations for the cloud
See what's new from DoiT in our latest news and announcements
Watch product demos, interviews and more from our cloud experts
Browse our open positions and learn more about what it takes to be a Do’er
Meet the team leading DoiT and our customers on a journey of hypergrowth
See what's new from DoiT in our latest news and announcements
Cloud cost savings from DoiT Flexsave enable Cloudify to reinvest in growing product portfolio
Cloudify provides customers with cloud orchestration and end-to-end network automation, extending their network and application services from their core location and making them accessible across branches and multi-access edge devices. The product, which works across public, private, hybrid and multi-cloud environments, can automate DevOps operations and support cloud migration efforts.
Cloudify’s SaaS product and services are built on complex, engineering-heavy development. From continuously improving their solutions to keeping up with the constantly changing public cloud architectures and replicating client issues in their own cloud environments, Cloudify depends on significant compute resources. This young, early stage innovator must guard their limited resources carefully, making sure not to waste money on over- or under-provisioning their public cloud compute and leveraging any available cost reduction opportunities.
Commitment-based discounts offer the richest savings available, but they require accurate forecasting over 1- and 3-year terms – practically eons for a small, growing start-up. Cloudify’s varied usage and need for flexibility across regions and machine types make it very difficult to do the forecasting necessary to take advantage of those commitments.
Cloudify was left to rely on manual management of compute discounts and all the forecasting that these required. This meant they didn’t always get maximum value from those efforts — usually because the commitments were tied to specific machine types that were deprecated or because the need for certain workloads was too unpredictable to commit to a set amount of money or usage. For a young, growing start-up company, this meant less money was available for reinvestment in the product, thus necessitating an automated solution that could optimize their compute costs without sacrificing their infrastructure flexibility.
After Flexsave was released, Cloudify jumped at the chance to take advantage of its ability to cover on-demand workloads in their AWS environment with rates equivalent to 1-year commitment discounts.
The company now realizes 20-30% savings each month on their EC2 spend. Additionally, they no longer have to spend several hours each week reconfiguring and rightsizing their compute workloads, or monitoring their commitments to ensure that they don’t exceed the amount covered by discounts.
Cloudify’s team structure is especially suited to this kind of flexibility of compute resources. At Cloudify, each developer and customer success manager has the autonomy to spin up new instances as needed, because they are able to operate quickly and independently without needing to worry about the expense of on-demand workloads.
As Cloudify grows their product portfolio and therefore their underlying cloud needs, Flexsave will continue to help keep their cloud costs optimized and automated.
20 Minutes reassigns two full-time engineers to new projects with the help of DoiT
Discover how Candid accelerated success with DoiT’s expertise. From optimizing AWS costs to enhancing cloud infrastructure, DoiT helped Candid innovate and thrive in dental care.
Leanspace pioneers modular satellite operations solutions, partnering with DoiT for cost optimization and infrastructure efficiency to propel its growth and innovation.
From cost optimization to cloud migration, machine learning and CloudOps,
we’re here to make the public cloud easy.
Ready to get started?